
Senior Engineering Manager, Google CoreML
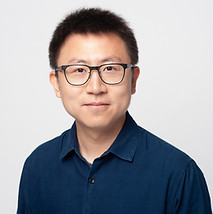
I lead ML/GenAI training infra team in Google CoreML to support product area such as Ads, Bard and Search.
Previously, I led data, ML infrastructure and applied ML teams in Project Starline - the next generation of telepresence at Google.
Before that, I founded the simulation team for Everyday Robots Project at X (formerly Google [x]). I built and managed cross geolocation teams with software engineers, researchers, and technical artists. I led the team to collaborate with Google Brain and DeepMind on a dozen research projects, including Sim2Real, and PaLM-SayCan.
I received my Ph.D. degree in Computer Science from Georgia Institute of Technology in 2015, under the advice of Dr. C. Karen Liu. My thesis focuses on designing algorithms for synthesizing human motion of object manipulation. I was a member of Computer Graphics Lab in Georgia Tech.
I received my B.E. degree from Tsinghua University, in 2010.
MY LATEST RESEARCH
We describe a system for deep reinforcement learning of robotic manipulation skills applied to a large-scale real-world task: sorting recyclables and trash in office buildings. Our system - RL at Scale (RLS) - combines scalable deep RL from real-world data with bootstrapping from training in simulation, and incorporates auxiliary inputs from existing computer vision systems as a way to boost generalization to novel objects, while retaining the benefits of end-to-end training.
The success of deep reinforcement learning (RL) and imitation learning (IL) in vision-based robotic manipulation typically hinges on the expense of large scale data collection. We introduce RetinaGAN, a generative adversarial network (GAN) approach to adapt simulated images to realistic ones with object-detection consistency. We show our method bridges the visual gap for three real world robot tasks: grasping, pushing, and door opening.